Email marketing remains, by and large, among the most promising marketing channels today – if not the king among them. Its excellent engagement rates, click-through rates (CTR), and response rates make for truly stellar Return On Investment (ROI) that some quantify as up to 4400%. In addition, more than 4 billion people already use email, and that number only increases over time.
Still, email marketers face challenges; open rates still average 21.5%, for instance. That’s indeed a 3.5% improvement over last year, but it still leaves room for improvement. Similarly, average email deliverability still sits at 84.2% – an improvement, but 15.8% of all emails remain lost.
Predictive sending seeks to address such challenges and help optimize your email marketing strategy. At its core, predictive sending uses machine learning and AI to analyze historical open rate data and deduce, or “predict,” the ideal sending times for future emails. In doing so, it overlaps with and facilitates behavioral email strategies, allowing for better open rates and more engagement.
However, that’s just a cursory glance at how predictive sending works and benefits campaigns, so let us delve deeper.
Why predictive sending is important
First, let us outline the benefits of predictive sending in more specific terms. Primarily, its key benefits are the three that follow.
#1 It enhances personalization
First, predictive sending directly enhances personalization. As highlighted in our behavioral email article above, different audience segments respond better to different delivery times. Thus, email timing can spearhead personalization alongside other practices like subject line and copy optimizations. As we’ll outline later, some days and times are universally better, but the point of segment-based personalization still stands.
Here, it is crucial to consider the advantages of this customer-first marketing philosophy. DigitalDot’s aggregated research finds it positively affects many key metrics, including:
- Open rates
- CTR
- Conversion rates
- Unsubscribe rates
- Retention rates
Retention rates, in particular, are also very noteworthy here since they drastically affect customer-related costs, as LinkedIn illustrates:
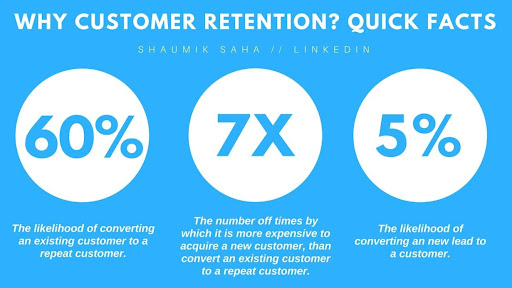
Should you need further information on the benefits of personalization, StartUpBonzai’s aggregated statistics also delve into them in-depth.
#2 It boosts open rates
On that subject, predictive sending most notably and substantially affects open rates. Beyond personalization, the way it does so is simple and lies in its fundamental function; learning from historical data.
To illustrate this, consider the following event sequence:
- During specific dates, recipients or segments produce specific open rates
- Predictive sending accounts for said rates and suggests the most historically effective time windows
- Based on new subsequent data, predictive sending adjusts and further refines its suggestions over time
This simple function yields open rate benefits, as it directly accounts for what individual recipients or segments prefer. In doing so, it effectively personalizes email timing further – and can of course synergize with event triggers.
#3 It enhances email deliverability
Third, successful email campaigns enhance your email deliverability in turn. This was one reason for citing these two metrics in the introduction; they somewhat overlap
The concept is fairly similar if you have experience with Search Engine Optimization (SEO). In SEO, engagement with content (alongside many other factors, granted) boosts one’s authoritativeness and thus fuels SEO. The same principle applies to email marketing; as predictive sending enhances your open rates, your sending authority increases. As your authority increases, your email deliverability improves. And as we’ve established, personalization does enhance open rates, as Oberlo also notes:
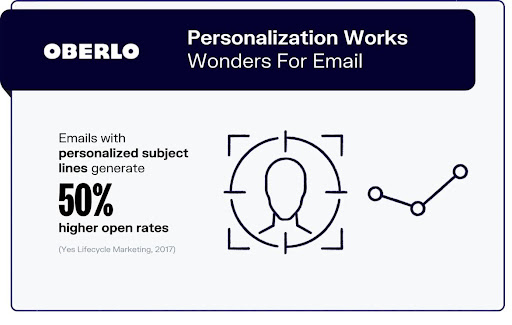
That said, just like SEO, email deliverability is a multifaceted challenge. So if you’d like a deeper analysis of this subject, you may also consult our article on improving email deliverability.
The impact of Mail Privacy Protection (MPP) on predictive sending
Finally, before exploring how predictive sending works in VBOUT and how you can leverage it, let us briefly address MPP. This development, cited in the above research as a potential challenge ahead, deserves an overview in this context.
What is Mail Privacy Protection?
First, let us define what MPP is. MPP is an opt-in feature for iOS, iPadOS, and macOS, seeking to protect users’ data from marketers. It was first announced when Apple unveiled iOS15 and will likely remain a staple iOS Mail app feature.
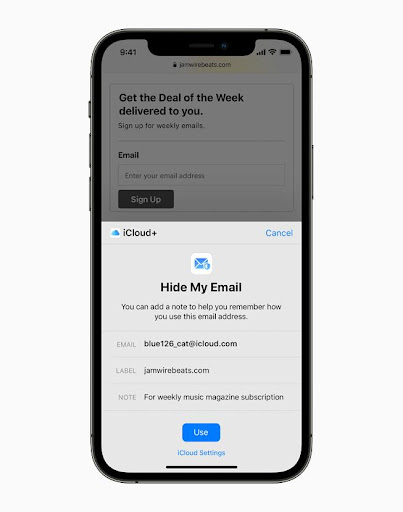
According to Apple’s announcement,
“[MPP] stops senders from using invisible pixels to collect information about the user. [It] helps users prevent senders from knowing when they open an email and masks their IP address so it can’t be linked to other online activity or used to determine their location.”
This direction coincides with other data protection initiatives, but MPP remains specifically noteworthy here.
How Mail Privacy Protection will affect predictive sending
That’s because MPP will indeed have an impact on predictive sending. We’re already observing this impact as more users opt into it. Its most notable effects are:
- Less accurate open rates, as it obscures them.
- Less reliable geographic targeting and segmentation, as masking IP addresses hinders them.
- Harder device-based segmentation since device and OS information is obscured as well.
There are, of course, solutions to this, which predictive sending itself will have to adopt over time. In our context, the primary one is to slowly shift to click rates as a metric to gauge success through. As MPP coincides with Google’s ongoing plans to phase out third-party cookies, another practice that will gain popularity is first-party data collection.
Still, MPP is not alone in setting email trends in the future. You may also consult our article on other notable trends and email marketing benchmarks for more on this broad subject.
How predictive sending works in VBOUT
Finally, in this section, let us outline how predictive sending works in VBOUT. Hopefully, doing so will give you a clearer idea of the practice on the whole and suggest ways you may integrate it into your email campaigns.
In brief, VBOUT initially offers a machine learning subject line checker within its email marketing feature. This checker also leverages the predictive sending concept by ensuring subject lines are optimal before all else. As established above, this element is crucial to personalization and open rates.
This checker looks as follows:

Next, as regards predictive sending itself, VBOUT offers three types of predictive sending that cover its three main forms. These are contact-based, business-type-based, and the fallback type.
What follows is, of course, just a cursory glance through them for the sake of text economy. If you’d like a deeper overview of VBOUT’s relevant features, you may consult our predictive sending help documentation.
#1 Contact-based
Contact-based offers the best-case scenario, where campaign emails are known contacts. In this case, historical data exists and can reliably inform outreach timing.
#2 Business type-based
Conversely, business type-based predictive sending caters to unknown contacts. The recipient’s business type is known in this scenario, so outreach timing can depend on it instead.
#3 Fallback
Finally, as the name suggests, the fallback type offers a fallback measure. In this scenario, the email is not a known contact, and the recipient’s business type is also unknown. In this case, predictive sending relies on optimal outreach timing based on thorough market research instead.
Conclusion
Predictive sending leverages machine learning to deduce ideal email outreach timing. Depending on the contact information and historical data available, it may primarily do so based on the recipients’ contact status, business type, or market research consensus.
In all cases, predictive sending offers an additional layer of personalization that lets marketers cater to individual recipients and segments. It can serve as an invaluable asset moving forward, especially in tandem with subject line optimizations, behavioral email marketing, and other personalization-minded optimizations.
About the author
Dylan Jacobson is a content writer and an avid SEO practitioner. He’s a regular contributor to Digital Dot, where he delves into the ever-changing SEO landscape, developments in email marketing, and the evolution of PPC. He enjoys the great outdoors and a night in town with friends and family in his spare time.
Don’t forget to share this article