Digital marketing has arguably been intensely competitive since its infancy. While competitiveness is nothing new to the field, technological innovations and emerging trends have only pronounced it in recent years. In response to the uncertainty that many businesses face due to this tumultuous landscape come predictive analytics. Far from a new practice in itself, predictive analysis offers to inform data-driven decisions based on outcome probabilities. Still, in such times of uncertainty, especially due to COVID-19’s lingering effects, it seems to enjoy newfound appeal. Thus, let us devote this article to exploring how predictive analytics can help improve your marketing strategy and ensure prosperity.
An introduction to predictive analytics
Starting with the definition, predictive analytics are very easy to broadly define – but the term also encompasses multiple different disciplines.
At their core, predictive analytics use data to fuel predictive models and predict future behaviors, results, and events. SAS offers an expanded but still consolidated definition of predictive models:
“Predictive models use known results to develop (or train) a model that can be used to predict values for different or new data. Modeling provides results in the form of predictions that represent a probability of the target variable (for example, revenue) based on estimated significance from a set of input variables”.
As such, based on either definition, they offer businesses the opportunity to act proactively and solidify their marketing funnels. This is arguably a key reason behind the predictive analytics industry’s exponential year-over-year growth, which Grand View Research quantifies as 23.2%.
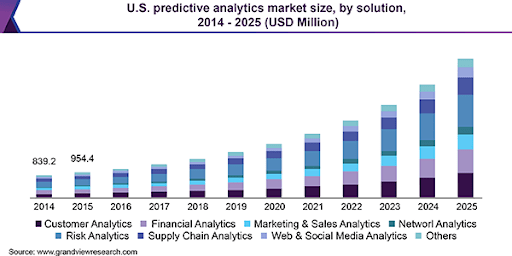
That said, there are multiple predictive analytics subdivisions to consider before delving into their practical applications. To only briefly outline the most substantive ones, consider the three that follow.
Types of business analytics by focus
First, as the graph above illustrates, different types of business analytics focus on different metrics. Some, like customer analytics, delve into customer data, in turn allowing for more precise improvements to the Customer Experience (CX). Others, like financial and risk analytics, delve into internal data and inform financial decisions.
Understandably, this precision allows for enhanced predictive accuracy and offers a foundation for differentiation among different predictive analytics solutions. To reiterate the graph, notable areas of focus include:
- Customer analytics
- Financial analytics
- Marketing and sales analytics
- Network analytics
- Risk analytics
- Supply chain analytics
- Web and social media analytics
Types of business analytics by function
In addition, there are different business analytics as regards to their function. The three most common ones are:
- Descriptive analytics. These analytics typically include historical company data and serve to best describe events that have transpired.
- Predictive analytics. The namesake type of predictive modeling, these analytics use algorithms to predict future events.
- Prescriptive analytics. Finally, these analytics deduce optimal courses of action based on the two prior types.
Adding a 4th subset of diagnostics, which some incorporate into descriptive analytics, SDG Group illustrates the use of each:
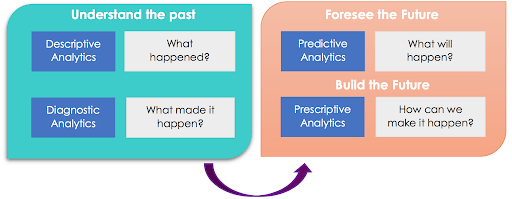
Types of predictive models
Finally, predictive analytics use different predictive models to best focus on individual marketing goals. The three most common ones are:
- Cluster models. These models use different variables to fuel customer segmentation. Examples of this include behavioral segmentation based on behavior data and value-based segmentation based on purchase history. Predictive analytics can help improve your marketing strategy by identifying and hyper-focusing on such customer groups through cluster modeling.
- Propensity models. Instead, propensity models focus on customers’ propensity to behave in specific ways – as the name implies. Through them, one can inform lead segmentation by predicting disengagement, churn, and so forth. Similarly, they can further inform value-based segmentation through predicted customer lifetime value (CLV).
- Collaborative/recommended filtering. Finally, these models evaluate past customer behavior and purchase history. This approach specifically helps identify new sales opportunities and typically focuses on cross-selling and upselling to existing customers.
Naturally, not all businesses will derive equal value from all of the above subdivisions. Rather, predictive analytics might best be perceived as solutions to specific problems or tools toward particular goals.
Practical applications: how predictive analytics can help improve your marketing strategy
With the above theoretical context in mind, we may now delve into practical applications of predictive analytics. In no particular order, consider the following five ways in which predictive analytics can help improve your marketing strategy.
#1 Audience segmentation
First, as highlighted above, predictive analytics facilitate more accurate and deeper audience segmentation. Through cluster models, businesses can initially segment audiences based on such factors as:
- Behavior; behavioral segmentation outlines shared behaviors among audience groups.
- Psychology; psychographic segmentation explores psychological drives such as peer influence.
- Value; value-based segmentation outlines CLV and informs lead scoring.
Then, they may use deeper predictive analytics to segment audiences further:
- Affinity analysis; this method fuses other segmentation methods, segmenting audiences based on shared attributes.
- Churn analysis; this method delves into churn rates and their potential impact on revenue.
- Response modeling; adjacent to psychographic segmentation, this method analyzes past audience responses to specific stimuli to predict future audience responses.
Understandably, these levels of audience insights can find ample use in one’s marketing strategies. In fact, in the context of marketing, they will serve as the bedrock of most practical applications of predictive analytics.
#2 Content marketing
An initial example of how predictive analytics can help improve your marketing strategy lies in content marketing on the whole. In this regard, consider such applications across content marketing endeavors as the following.
Search Engine Optimization (SEO)
Understanding audiences’ interests and behaviors can meaningfully inform keyword research and help match content to users’ search intent throughout their journey. Moreover, especially combined with web analytics, it can inform other copywriting factors that affect engagement, from content length to formatting. In conjunction, these improvements can enhance search engine ranking and, in turn, online visibility for higher lead generation.
Social media marketing
In much the same way, predictive data can inform social media activities as SEO and social media go hand in hand. For example, response modeling can help identify ideal engagement times, copy, and so forth based on response history. In tandem with such social media audience tools as Facebook’s Audience Insights and a healthy SEO foundation, predictive analytics can elevate lead acquisition to new heights.
Paid advertising
Finally, all of the above can also inform paid advertising campaigns such as Pay Per Click (PPC) ads. Deep customer analytics can identify optimal advertising channels, outreach timing, and other critical marketing factors. In turn, paid advertising can target audiences SEO and social media cannot, in cases where, for example, desirable keywords are too competitive to organically rank for.
#3 Customer acquisition and retention
Following the above, predictive analytics help facilitate the increasingly popular flywheel model, which HubSpot explains hinges on attracting and retaining customers through a consistently pleasing UX:
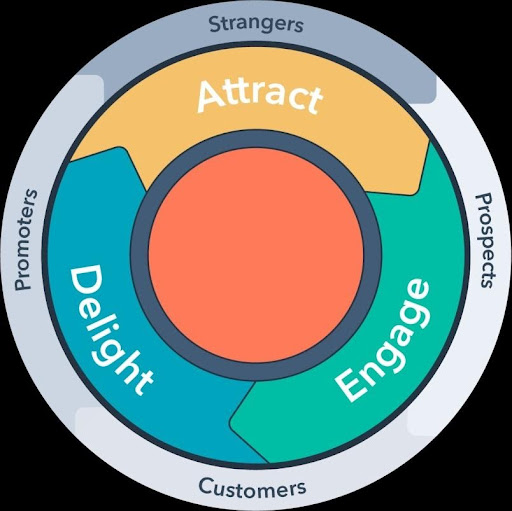
In this context, then, “attract” equates with customer acquisition, while “engage” and “delight” broadly equate to retention.
To address both, we may first reiterate some points mentioned above. In brief, predictive analytics facilitate:
- Increased online visibility. Through optimal advertising channel choices and healthy SEO, audiences may find you more easily.
- Better search intent match. With deep customer insights in hand, you may update your landing pages to offer more engaging content that helps acquire generated leads.
- A personalized UX. Finally, through a personalized customer journey, audiences remain delighted and become more likely to convert.
Then, we may pinpoint such practices as email marketing. Indeed, email marketing efficiency is one of the key ways in which predictive analytics can help improve your marketing strategy.
To illustrate this, we may initially note the benefits of AI, a core component of predictive analytics, in email marketing, as outlined by Statista:
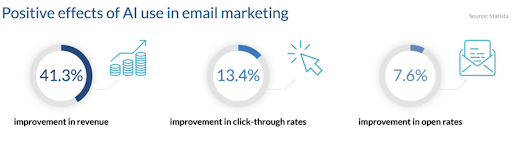
Then, we may note that email marketing, from automation to personalization, benefits significantly from Customer Relationship Management (CRM) software. It is perhaps for this reason that, as Statista notes, CRM is currently the largest software market in the world:
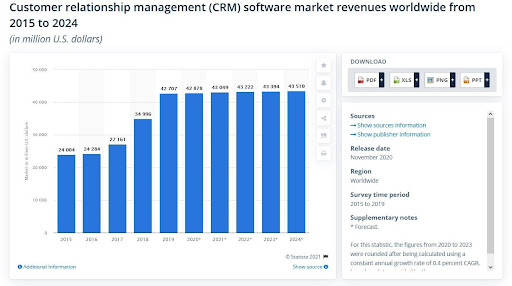
Thus, we may argue that email marketing remains immensely valuable between relative ease of implementation and demonstrable cost-efficiency. In turn, predictive analytics can augment it further through data-driven copy optimizations, personalization, and outreach timing. Especially in conjunction with CRM’s automation capabilities, email campaigns with such qualities can spearhead retention strategies.
#4 Upselling and cross-selling
Having touched on email marketing, we may then address upselling and cross-selling. While exact predictive analytics applications in other areas may vary, this application is the explicit goal of collaborative/recommended filtering.
Brand loyalty and advocacy aside, customer retention is so valuable because it’s demonstrably lucrative. Indeed, according to AnnexCloud, “[a] 5% increase in customer retention can boost profits by 25% to 95%”. That’s in no small part because “[a]lmost 65% of a company’s business comes from repeat customers[, and] 82% of companies agree that retention is cheaper than acquisition.”
These findings can be used as regards upselling and cross-selling, as these practices address existing customers. Thus, the way predictive analytics can help improve your marketing strategy in this regard is twofold; first, they enhance retention rates, and then they reveal opportunities to leverage said retention rates. By analyzing customer behaviors, purchase histories, and CLVs, you may identify such opportunities through collaborative/recommended filtering. Then, you may use these insights to inform social media campaigns and email campaigns, presenting customers with optimally priced, relevant propositions at opportune times to boost sales.
#5 Improved regression models
Finally, to enhance ongoing marketing strategies and inform future ones, you may use predictive analytics to create improved regression models. Indeed, Tibco finds that regression models are perceived as notably valuable:
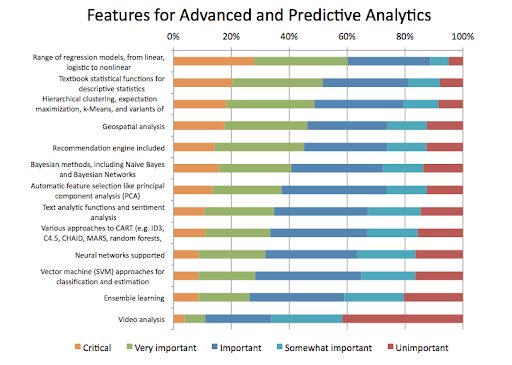
Understandably, regression analysis can also see a wealth of marketing applications. Should you not be using it already, Driveresearch provides the following definition:
“Regression analysis is a common technique in market research which helps the analyst understand the relationship of independent variables to a dependent variable.”
Among the most common applications of regression analysis, as Driveresearch also notes, is Net Promoter Score (NPS) monitoring. That is, it explores how the dependent variable of NPS advocacy changes depending on different marketing variables. This specific example aside, however, regression analysis can be employed throughout marketing strategies, wherever variable relationships are valuable.
Conclusion
To summarize, predictive models offer to predict future outcomes with increasing accuracy. Thus, predictive analytics can help improve your marketing strategy from the initial step of customer segmentation to the final stage of campaign monitoring. Through deep audience insights and historical data, they can solidify your SEO, social media campaigns, and email campaigns. In turn, they can facilitate better customer acquisition and retention rates, holistically improving the customer journey and UX. Finally, they can seamlessly integrate into your existing operations through significant overlap with CRM, email automation software, and regression analysis. Predictive analytics can be an invaluable marketing asset, from slight marketing optimizations to the complete adoption of the flywheel model.
About the author
Andrew Johnson is a copywriter and web developer based in NYC. He frequently collaborates with Movers Development, for whom he authors articles on emerging digital marketing trends, innovative technologies, and news specific to the relocation industry. When he doesn’t fervently work on new content, he studies for his MSc in Zoology.
Don’t forget to share this article